Building an Optimized ML Pipeline
Qwak and members of Happening's data science and ML engineering teams discuss how they built Superbet's profanity detection model.
During this session we will discuss:
- What best practices have been implemented for optimizing MLOps workflows and reducing operational efforts when re-deploying ML models.
- How the team ensured fallback variations for multi-language models without duplicating efforts
- How all this was implemented in Superbet's profanity model architecture, designed to identify profane messages in chat messages.
This session aims to provide actionable insights into how you can optimize your ML pipeline based on the team's experience.
If you're a data scientist, ML engineer, or anyone interested in learning how to optimize ML pipelines for multiple audiences, this webinar is for you. Don't miss this opportunity to learn from the experts at Happening and improve your ML pipeline.
Next Event
Qwak and members of Happening's data science and ML engineering teams discuss how they built Superbet's profanity detection model.
During this session we will discuss:
- What best practices have been implemented for optimizing MLOps workflows and reducing operational efforts when re-deploying ML models.
- How the team ensured fallback variations for multi-language models without duplicating efforts
- How all this was implemented in Superbet's profanity model architecture, designed to identify profane messages in chat messages.
This session aims to provide actionable insights into how you can optimize your ML pipeline based on the team's experience.
If you're a data scientist, ML engineer, or anyone interested in learning how to optimize ML pipelines for multiple audiences, this webinar is for you. Don't miss this opportunity to learn from the experts at Happening and improve your ML pipeline.
Learn more from top AI experts
Qwak optimizes AI in production
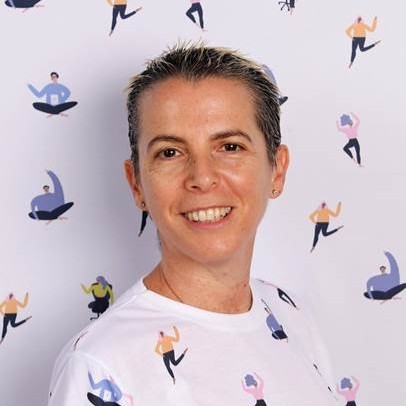
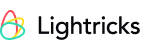
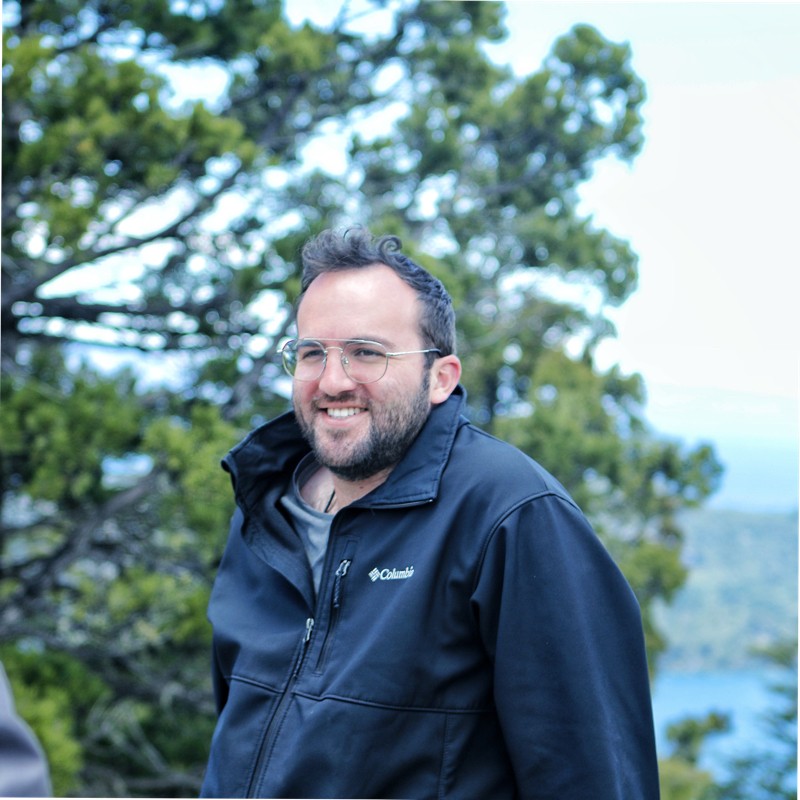

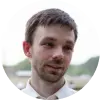
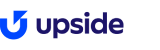