Generative AI at Scale with Enhanced Data Capabilities

Delivering generative AI in production
The partnership between Snowflake and Qwak offers seamless, efficient, and scalable solutions for generative AI. Businesses can leverage both platforms for improved data management, real-time updates, and advanced model fine-tuning. There are multiple touchpoints and potential applications where the integration between Snowflake and Qwak can be leveraged. In this blog post, we'll explore the various available options in the journey of bringing generative AI to production.
Integrating Snowflake and Qwak
Integrating Snowflake and Qwak offers numerous advantages for deploying Large Language Models (LLMs) and other generative AI applications. This synergy allows the creation of robust, scalable solutions that can handle complex data processing and model deployment tasks. By leveraging Snowflake's data management capabilities alongside Qwak's end to end AI platform, businesses can achieve real-time data updates, seamless model fine-tuning, and efficient version control.
- GenAI Applications: The integration supports the deployment of generative AI models that enable applications such as chatbots, automated content creation, and personalized user experiences. With continuous data ingestion pipelines and model fine-tuning, these models remain highly accurate and contextually relevant.
- RAG Retrieval: Utilizing Qwak's Vector Store for embedding models and Snowflake's robust data storage, businesses can optimize LLM applications through Retrieval-Augmented Generation (RAG) techniques, ensuring faster, more accurate responses by continually refining the data fed into the models.
- Ongoing Maintenance: This integrated approach not only enhances the AI model's capabilities but also streamlines the entire AI lifecycle, from data ingestion to model deployment and monitoring, significantly improving operational efficiency and reducing time-to-market for generative AI solution.
Seamless data ingestion
- Scheduled Data Ingestion: Prepare features both for model training and model inference from your Snowflake warehouse using the feature store schedules ingestion features.
- Vector Store Updates: Easily transfer data from Snowflake to Qwak’s Vector Store using embedding models deployed on Qwak. This integration supports continuous data updates, ensuring that the Vector Store remains up-to-date, critical for optimizing Large Language Models (LLM) and Retrieval-Augmented Generation (RAG) applications.
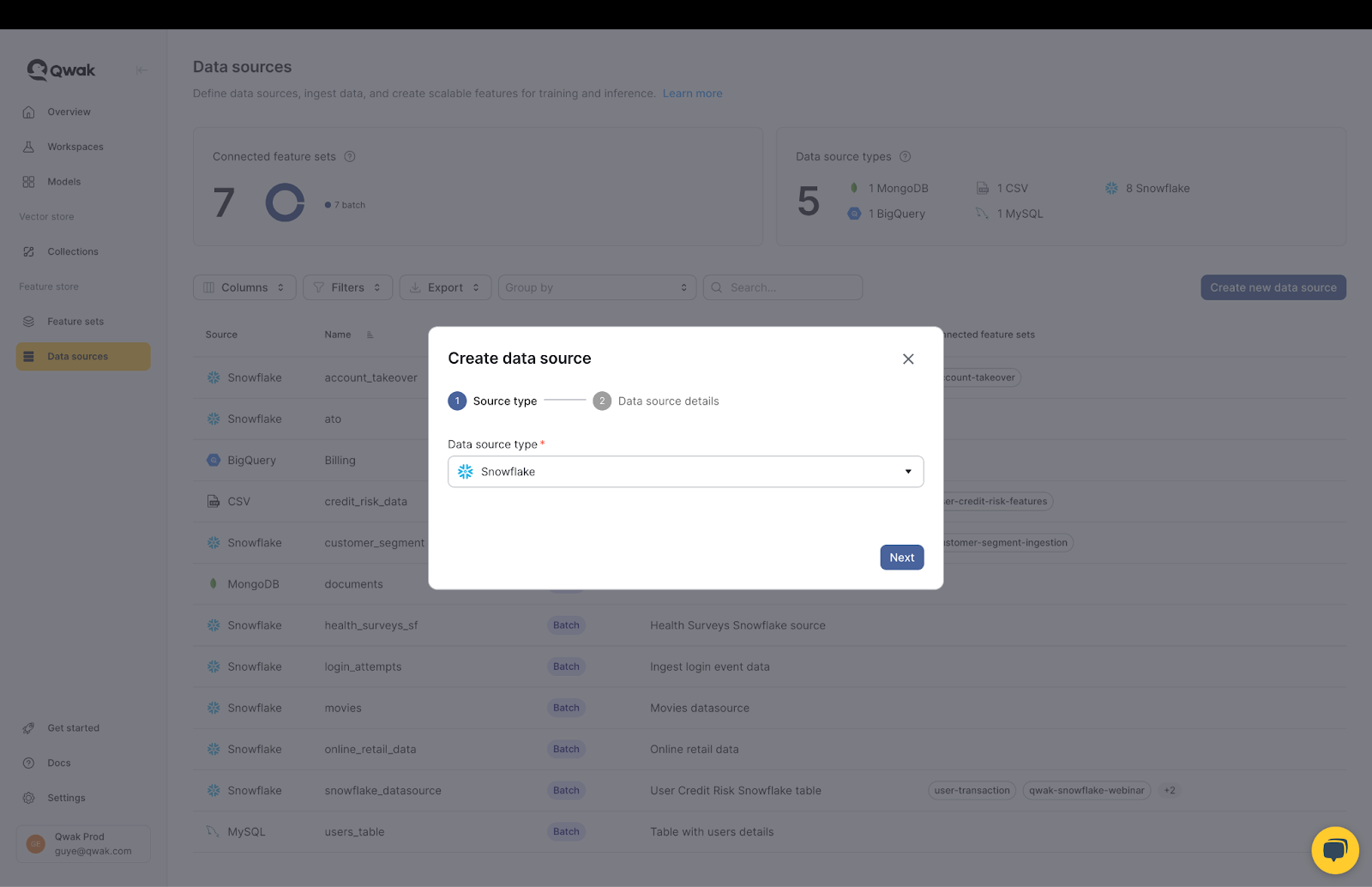
Feature store integration
- Real-Time Feature Updates: Move data dynamically from Snowflake to Qwak's feature store. This integration is key for maintaining fresh data, which are essential for minimizing response times in generative AI applications and reducing overall inference latency.
- Enhanced Data Features: Access enriched data features that are continually updated, ensuring that your AI applications perform at peak efficiency with the most current data available.
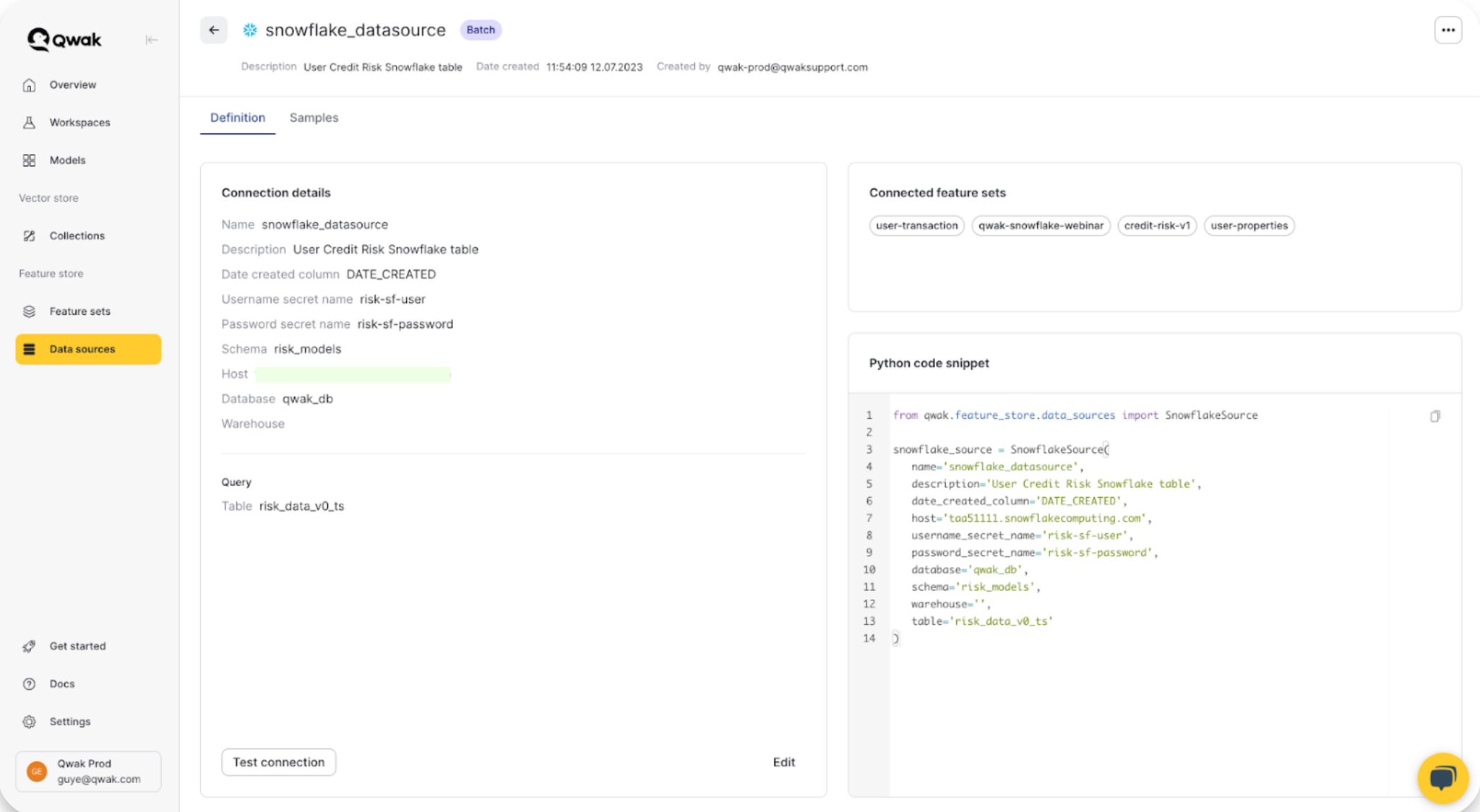
Model fine-tuning
- Access Datasets: Use Snowflake's storage capabilities to maintain and manage large datasets used for model fine-tuning on Qwak. This allows for seamless access to datasets of any size
- Version Control: Efficiently manage model versions within Qwak’s model registry, ensuring that model versions are tracked and deployed without disrupting existing applications.
Model inference analytics
- Centralized Data Management: Integrate the Qwak Analytics Lake with Snowflake to store all model inference calls. This enables centralized management and analysis of inference data, providing insights into model performance and usage patterns.
- Analytical Insights: Gain valuable insights from stored inference data to continually refine and enhance model performance, ensuring that your generative AI applications adapt to evolving data trends and user needs.
Digital Banking Service - customer case study
Launching Banking ChatBot
Digital Banking Service sought to enhance their customer service capabilities by upgrading their existing chatbot, to a more advanced version. The objective was to create a chatbot that could handle more complex customer inquiries with higher accuracy and personalized responses.
Solution
Utilizing the partnership between Snowflake data cloud and Qwak, Digital Banking Service integrated their data systems with these platforms to leverage enhanced AI capabilities for Banking ChatBot. Below is a summary of the integration workflow and its impact:
- Data Integration: Digital Banking Service used Snowflake to manage and securely store vast amounts of financial data, which was then seamlessly ingested into Qwak's platforms for real-time processing and analysis.
- Feature Store and Vector Store Updates: Real-time data from Snowflake was continually updated in Qwak’s feature store and Vector Store, providing Banking ChatBot with the latest customer and financial data to ensure accurate and contextually relevant interactions.
- Model Fine-Tuning and Deployment: Utilizing the robust dataset available in Snowflake, The Digital Banking service fine-tuned the ChatBot’s AI model on Qwak, leveraging Qwak's model registry for efficient version control and deployment.
Outcomes
- Enhanced Customer Interaction: Banking ChatBot can now handle a wider range of queries with greater precision, leading to improved customer satisfaction rates.
- Operational Efficiency: The integration allowed for faster response times and more personalized communication, significantly reducing the workload on human customer service representatives.
Discover the full story
Read more about the Digital bank's journey and learn about their experience building a private banking chatbot.