

Feature | ![]() | ![]() |
---|---|---|
Zero-config model build & deploy | X | X |
Data source integration | AWS data sources | Multiple data sources |
Multi-cloud support | AWS | AWS, GCP, Azure |
Intuitive UI | X | X |
Support | Standard AWS support | Standard |
SageMaker, though powerful, demands a solid grasp of AWS and engineering expertise. Its UI is less intuitive than specialized platforms, requiring navigation and expertise through multiple AWS services.
Databricks leverages open-source tools like Apache Spark, MLflow and Airflow, which offer a lot of configurability but can be complex for some users. While it provides a robust set of features for big data analytics, it may lack specific out-of-the-box ML features, requiring users to build custom solutions using Spark. This adds a layer of complexity and requires a deeper understanding of the underlying technologies.
Feature | ![]() | ![]() |
---|---|---|
Model build system | X | Engineers required |
Model deployment & serving | V | V |
Real-time model endpoints | Engineers required | V |
Model auto scaling | Engineers required | Engineers required |
Model A/B deployments | Engineers required | Engineers required |
Inference analytics | Engineers required | Engineers required |
Managed notebooks | V | V |
Automatic model retraining | Engineers required | Engineers required |
SageMaker does not have Training Jobs or simple deployment, and its Experiments feature and Studio IDE introduce complexity. The deployment and monitoring processes entail manual engineering setup, with limited out-of-the-box support.
Databricks, a cloud-based platform integrating with various providers, heavily relies on Apache Spark for data processing. While it manages some infrastructure aspects, users need a good grasp of Spark configurations. In contrast. Databricks' deployment time varies; simple models can take minutes, but complex scenarios may extend to days, particularly without prior Spark experience. This variability, while enhancing flexibility, introduces complexity impacting deployment speed.
Feature | ![]() | ![]() |
---|---|---|
Managed feature store | V | V |
Vector database | V | V |
Batch features | Engineers required | V |
Realtime features | Engineers required | V |
Streaming features | Engineers required | V |
Streaming aggregation features | X | Engineers required |
Online and offline store auto sync | X | Engineers required |
The AWS Sagemaker Feature Store requires manual setup for feature processes and lacks support for streaming aggregations, necessitating additional services like Elasticsearch, Chorma, Pinecone, and others for similar functionality.
Databricks offers a Feature Store that supports batch data sources and allows for feature transformations using Spark SQL or PySpark functions. Features can be stored in both an Offline and Online Store but require manual schema definition. While it supports a range of data sources, it is optimized for the Databricks ecosystem. Streaming data sources and streaming aggregations are not natively supported.
Don’t just take our word for it
Qwak was brought onboard to enhance Lightricks' existing machine learning operations. Their MLOps was originally concentrated around image analysis with a focus on enabling fast delivery of complex tabular models.
Read Case Study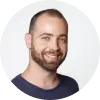
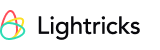