Top 13 ML Data Labeling Tools and Software in 2024

In the world of machine learning, data labeling is absolutely essential. After all, in order for a machine to learn from data, that data needs to be properly labeled. Without high-quality labels, ML models can't accurately learn from data and they won't be able to make reliable predictions.
The good news is that there are now tools that can automate the data labeling process, making it faster and easier than ever to get high-quality labels. ML data labeling tools use a combination of human reviewers and artificial intelligence to quickly label large amounts of data. This ensures that labels are accurate and consistent, and it frees up human reviewers to label more complex data.
In this article, we'll give you a rundown of everything you need to know about data labeling, including what it is, why it's important, and some of the top machine learning labeling platforms available. By the end, you'll have a good understanding of how to choose the right tool for your organization. So let's get started!
What is ML Data Labeling?
ML data labeling is the process of assigning labels to data so that machine learning algorithms can use it. This process usually involves adding tags or annotations to data points so they can be classified according to specific categories.
Machine learning labeling also offers a variety of features, including point-and-click labeling, keyboard shortcuts, bulk labeling, and integration with the Google Cloud Platform. This can be done manually or automatically by algorithms. Although there are other ways to facilitate machine learning labeling, the choice of method typically depends on the type of data and its intended use.

What are Data Labeling Tools?
As we saw, data labeling can be a time-consuming process when done manually. However, automating the process can streamline the entire thing.
This is where data labeling tools come into the picture!
These tools annotate or label data for various purposes, such as training machine learning models. Some common data labeling tasks include facial recognition, object detection, and labeling images and classification.
Depending on the tasks, you can choose from different types of data labeling tools available. These range from simple online annotation platforms to sophisticated software suites for enterprise-level annotation projects. However, make sure to consider the specific needs of your project and the level of complexity involved.
Top Data Labeling Tools
If you have been considering using data labeling tools for your project, you might want to have a sneak peek into the best tools available around.
So, here we go. Following ahead, we curated a list of the top 13 data labeling tools that have recently been quite popular. Let's look at each of them in a bit more detail considering their pros and cons.
1. Label Studio
Owned by Heartex, Label Studio is one of the most popular data labeling tools that can be used for a variety of tasks, including image classification, object detection, and semantic segmentation. It offers a user-friendly interface that makes it easy to label data sets of all sizes.
Pros
- Since it is an open-source tool, any user can download it for free;
- Quickly configurable for different data types;
- Simple interface;
Cons
- Prone to security attacks due to its open-source nature;
- Does not offer role-based access control for automatic data labeling in community mode;
- No support for single sign-on with LDAP or SAML;
2. Amazon SageMaker Ground Truth
Amazon SageMaker Ground Truth is a fully managed service that makes machine learning labeling easy. With Ground Truth, you can label data yourself or have Amazon Mechanical Turk workers label your data for you.
Moreover, it provides accelerated data processing for faster outputs and learnings and Kubernetes integration for complex deployments. Overall, it is one of the best data labeling tools for programmers who want to deploy machine learning models at any scale.
Pros
- Easy to manage and develop any given script;
- You are charged only for what and how long you use it;
- You can choose multiple servers for training without worrying about distribution;
- Training, testing, and models are easy to access whenever required;
Cons
- Somewhat complex for beginners;
- Heavy dependence on new releases;
3. Sloth
Sloth is another popular open-source data labeling tool mainly used for labeling images and videos for computer vision research. Users can access this platform's dynamic tools for data labeling in computer vision. Sloth is also popular because it easily lets you build your custom configuration or deploy your figures for data labeling. Therefore, if creating a custom configuration is your top priority, make sure to check out Sloth.
Pros
- You can also factorize and construct your own visualization items, which is a plus point considering the flexibility;
- You can also manage installation, labeling, and create customized visualization data sets;
- It also offers customizable dynamic tools you can use for data labeling;
- Easy to use because it simplifies the process of labeling images and video based data;.
Cons
- Although the interface is simple, it may be hard for beginners as some options are not straightforwardly accessible;
4. Tagtog
Tagtog is another common name among the most popular text-based data labeling tools specifically curated for text formats and activities to help build custom datasets for text-based tasks. It is primarily used as a text annotation tool, but you can also use it to manage the text labeling process and further enhance the processing speed.
Pros
- It is a user-friendly tool with a simple interface, which makes it easy to use;
- You can also access in-depth insights from text using the application;
Cons
- Since it is a text annotation tool specifically for NLP, it may be irrelevant for other tasks;
5. LightTag
Similar to Tagtog, LightTag is also another worthy addition to our list of most prominent text-based data labeling tools, designed specifically to perform automatic data labeling for NLP data sets. While using this tool, ML teams can expect super efficient collaborative workflow, making it a popular choice among its users.
Pros
- Since it is deployed on the cloud, it can be easily accessible independent of location and perform automatic data labeling;
- You can handle data perfection as the annotators work perfectly;
- The AI swiftly and just itself to the predictions, which provides a similar experience;
Cons
- It does not support audio files;
- You cannot access training data management;
- It does not support performance tracking;
6. Audino
When it comes to speech and audio annotation, Audino is clearly a top-notch choice among its users. It is a highly collaborative open-source tool to define and enable temporal segmentation for audio files, making it a popular choice among other labeling tools.
Audino can be an ideal tool if you are looking for better flexibility as an admin because it lets you take complete control of the user roles and project assignments accessible through the dashboard. In addition, it comes with a key-based API, enabling you to upload and assign audio data to respective users.
Pros
- It comes in a dynamic form, making it simple to use and transcript the portions;
- Extensive flexibility allows you to annotate speaker identification, characterization, speech recognition, emotion, and voice activity detection tasks;
- It can be used for professional and academic purposes, as it comes with an open-source license;
Cons
- It may look complicated for complete beginners;
7. Dataloop
Data loop is a popular end-to-end automatic data labeling platform covering all processes from development to production. It has been developed keeping in mind the essence of data-centric technology that includes annotation and data management to streamline data generation for deep learning.
It also provides excellent support for easy handling and simplifying operability details, making it a significant preference for case-specific tasks. If you primarily work on computer vision projects for production and are looking forward to extensive engineering, Dataloop is an ideal choice for your projects!
Pros
- You can execute your open-source packages as services on different compute node types;
- It also allows the execution of combined services and enables adding new people or tasks for a given project;
- Excellent support for administration, especially for extensive hours;
Cons
- It may become expensive in the future as its pricing has gone up significantly in a short time;
8. Supervisely
Supervisely is another powerful AI-powered machine learning labeling tool that offers a wide range of features to help you label your data quickly and accurately. It is a fully self-hosted, cloud-based computer vision platform with an integrated API and SDK that can source codes. With Supervisely, labeling images becomes extremely easy and streamlined.
Pros
- Easy integration to different open source tools and custom-built solutions within a single ecosystem;
- Users can use the portfolio and connect with different interactive web apps powered by Python;
- It allows the capacity to deploy unlimited modifications and flexibility, a significant plus point keeping in mind the necessity to tackle any complexity in automatic data labeling;
Cons
- It may slow down after working for a while;
- It can be overwhelming for new users looking forward to working with the program as they may need help in fully understanding its functions and how to implement them for labeling images;
9. Doccano
Doccano is an end-to-end text open-source annotation tool that makes it easy to train and deploy your own custom models to facilitate automatic data labeling. It supports various classification tasks, including sentiment analysis, topic classification, spam detection, and more. With Doccano, you can easily train and evaluate your models with a few clicks.
Pros
- It has extensive features for labeling images such as sequence labeling, named entity identification, and text summarizing;
- This tool is really great with speed;
- It comes with a collaborative annotation, supports multiple languages, and allows Smartphone and emoji compatibility;
Cons
- It does not support dynamic processes for specific tasks such as labeling images;
- The implementation process can become tricky due to security issues, as it might require an external API. This would need you to proceed with launching an external server;
10. Labelbox
Another powerful addition, Labelbox, is a machine learning labeling tool that helps you create labels for your data. This is useful when you categorize or organize your data for later use. Labelbox is precisely engineered to help you improve your training data, data annotation, and model performance diagnosis. It allows you to create custom labels and automatically generate labels based on specific criteria.
If we talk about its features, it comes with highly collaborative functionalities to help you collaborate efficiently between labelers and multiple domain experts for labeling images.
Pros
- It helps you lower annotation costs by 50% by leveraging machine learning labeling automation and active learning;
- It enables iteration three times faster on your artificial intelligence data to help you build more performance-oriented models;
Cons
- You might face issues labeling images for scientific imaging tasks;
- The focus on unique IDs may feel irrelevant as it is easy to identify your data by their titles;
11. V7
It is one of the most extensively preferred and powerful data training platforms specifically for computer vision that brings together dataset management, image, and video annotation, and machine learning model training. It also helps you to store, annotate, manage, and enable automatic data labeling and annotation workflows across various file formats.
Pros
- It is very easy to manage images, annotators, and annotations;
- Easy workflow management and quality control stages to streamline automatic data labeling;
- It also comes with an auto annotation feature, which makes it a strong preference among annotators;
- Extensive and highly responsive support team;
Cons
- You might experience occasional lagging while working with large data sets;
- There is no option to pitch in an image searching pane for the users, which may be necessary for specific tasks;
- Vocational sign-out can be another potential annoyance;
- The platform does not allow access to workers' stats on a project even after exporting data;
- The documentation may feel somewhat rough on the edges;
12. Lionbridge AI
Another cutting-edge addition to the countdown, Lionbridge AI is easily among the best end-to-end data labeling tools usually preferred by data scientists working on training machine learning models.
Its AI offerings include training data, adaptive testing, and natural language processing. These applications also support some of the most common file formats, including text, audio, image, and video. The company has over 20 years of experience in the industry and works with some of the largest brands in the world, making it a reputable and trusted machine learning labeling product.
Pros
- It enables you to quickly create customized training data sets while maintaining efficiency and affordability;
- It also helps you maintain data integrity while working on projects;
- You can have complete control and flexibility to customize your assignment, quality checks, and workflow while working on critical machine learning labeling processes;
Cons
- Pricing can be an issue since it may not be affordable for small-scale organizations;
13. Superannotate
Superannotate is another leading AI-powered image annotation tool that makes it easy to facilitate labeling images for training machine learning models. It also helps you build high-quality training data sets for computer vision and NPL. This tool comes with advanced premium tools such as data curation, robust SDK, offline access, QA, and integrated annotation services.
It is one of the top-performing labeling tools that are well known to provide incredibly precise data sets and efficient pipelines up to 5 times faster than the regular ones. All in all, it is an excellent choice as an integrated software that gives you the ultimate service and experience in creating super-efficient data pipelines!
Pros
- If you’re looking for the best labeling tools, you can go for this one as it is also helpful for vector data, especially if you want your data to be annotated with precision and speed;
- The API is easy to use and gives the flexibility to enable you to develop a novel pipeline;
- The platform is user-friendly and has all the features needed to annotate unstructured data;
Cons
- It does not allow you to add classes while annotating and labeling images since they come in a pre-fixed format;
- More than specific annotation projects are quite open vocabulary, which may become inconvenient in the long term;
- The video segmentation pipeline does not come with tracking algorithms which may make it difficult to annotate automatically;
How to Choose the Right Data Labeling Tool?
As companies increasingly recognize the value of data in driving vital business decisions, the need for efficient labeling tools is also on the rise.
But with so many different options available, how do you choose the right one for your needs?
Here are some factors to consider when evaluating data labeling tools:
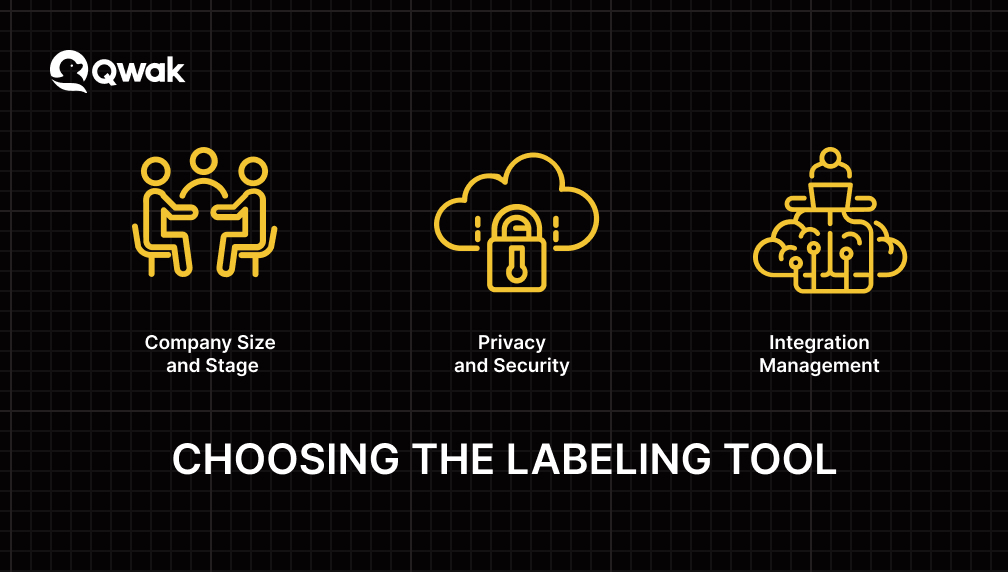
Company Size and Stage
The size of your company or the stage it's at in its development can play a significant role in which machine learning labeling tool is right for you. If you're a small startup, you may need more money for a costly enterprise solution.
On the other hand, if you're a large company with complex data needs, you will need more advanced labeling tools with robust enterprise solutions. Smaller companies or those in the early stages of development should look for more affordable and user-friendly solutions.
Privacy and Security
When it comes to data labeling tools, privacy and security must always be one of your priorities. That’s why choosing a data labeling tool with built-in privacy and security features is essential. Look for one that offers role-based access control. This ensures only those with the appropriate permissions can access your data.
Another essential security feature to look for is end-to-end encryption. Your data is encrypted in transit, and at rest, so unauthorized individuals can’t intercept or access it. Finally, keep in mind that ideal data labeling tools offer auditing and logging features. This way, you can track who accessed your data and when and ensure that all activity complies with your internal policies.
Integration Management
Integration management refers to planning, monitoring, and executing medium to large-scale changes to your IT infrastructure. It is a necessary factor to consider while choosing the right tool for automatic data labeling.
When making changes to your IT infrastructure, it is essential to consider how those changes will impact your data labeling processes. Some automatic data labeling tools are better suited for specific changes than others. For example, if you plan on adding a new data center, you will need a data labeling tool that can quickly and efficiently handle the increased volume of data.
Why Should You Choose Qwak?
Today, most practical machine learning models use supervised learning, which applies an algorithm to map one input to one output. However, for supervised learning to function, you need a labeled data set that the model can learn to make precise and accurate decisions.
Data labeling typically starts by asking humans to judge a piece of unlabeled data. For example, labelers may be asked to tag all the images in a dataset where “Does the photo contain traffic lights” is true. Here, the tagging can be anything from a simple yes/no to identifying the specific pixels in the image associated with the traffic lights.
The machine learning model uses human-provided labels to learn the underlying patterns in a process called "Model Training." The result is a trained model that can predict new data.
And when it comes to machine learning models, it's essential to understand that simplicity is critical for productionisation. Qwak understands this necessity and facilitates a platform that simplifies the productionization of machine learning models at scale. Qwak's powerful ML Platform empowers data science and ML engineering teams to deliver ML models for quick and efficient production.
By removing all the complexities of model deployment, integration, and optimization, Qwak streamlines and brings high velocity to all ML initiatives designed to transform business, innovate, and give you the right edge!

Unmatched Effort Deliverables Ratio
One of the biggest challenges developers face is the humongous time and effort that goes into aligning the setup between data science and engineering. Unfortunately, it has always been a prevalent issue and often leads to unpredictable delays causing irrevocable inconvenience!
But don't worry, we've got you covered! 🙌
With Qwak, you get access to the fastest ML-to-production life cycle, which completely eliminates this need for alignment. The setup lets you go through only the necessary steps, ultimately removing all the hassle!
No Endless Integration Efforts
Qwak is designed to minimize the integration effort required to use it within your organization. It includes out-of-the-box integrations with super-efficient build tools and dependency managers so you can get started quickly and easily.
We also provide a flexible system that offers multiple deployment options, allowing you to get the right fit for your specific project needs.
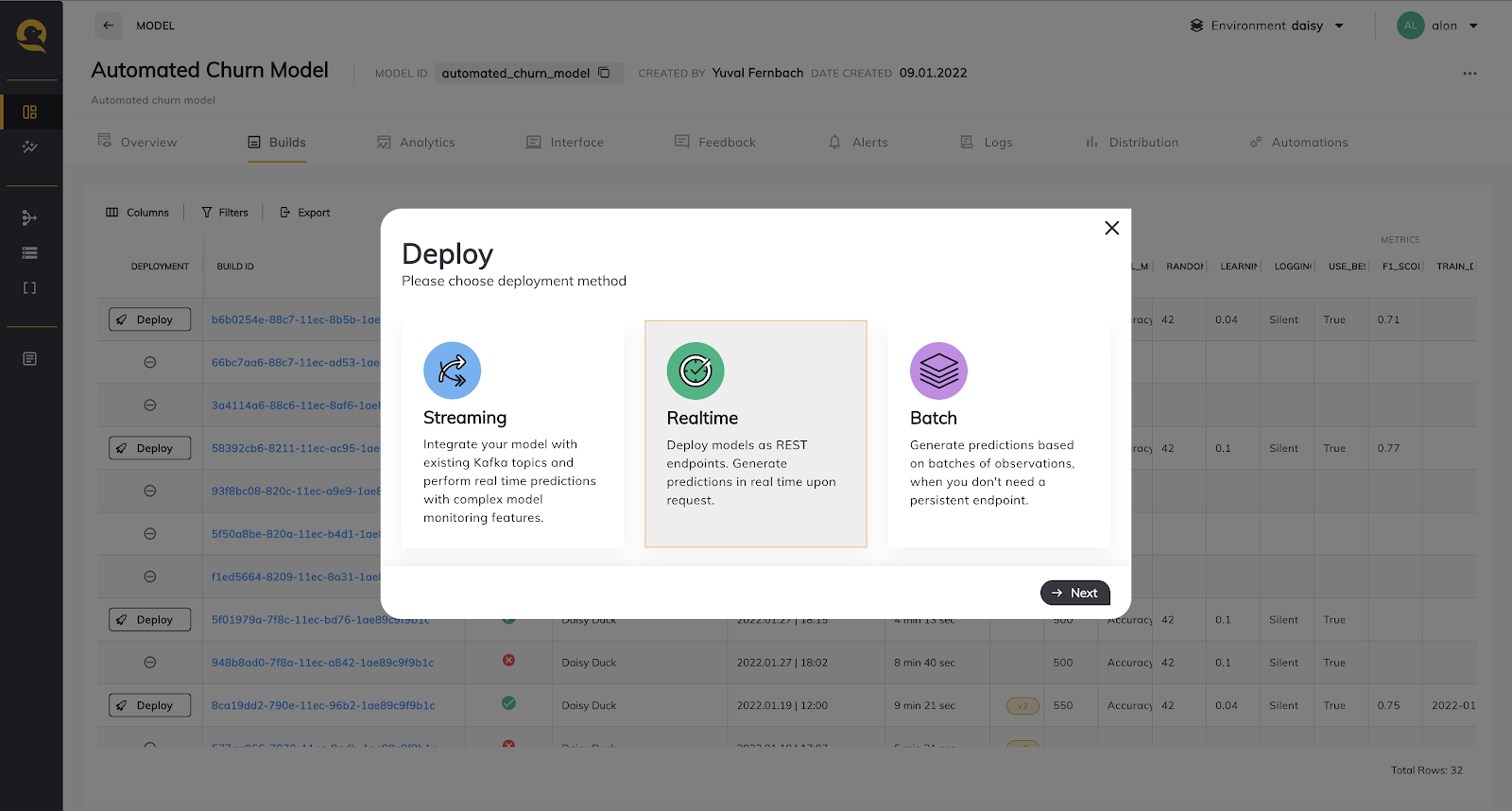
Click here to discover how Qwak streamlines ML-driven production for your projects!
Takeaways
Machine learning data labeling is a process that enables us to provide input for teaching and improving ML models. However, with a touch of automation, data labeling tools make it possible for businesses to cost-effectively outsource this work to a large, global workforce of labelers.
Qwak simplifies the productionization of machine learning models at scale. Qwak’s Feature Store and ML Platform empower data science and ML engineering teams to Build, Train and Deploy ML models to production continuously.
By abstracting the complexities of model deployment, integration, and optimization, Qwak brings agility and high velocity to all ML initiatives designed to transform business, innovate, and create a competitive advantage.