Unlocking business value with machine learning
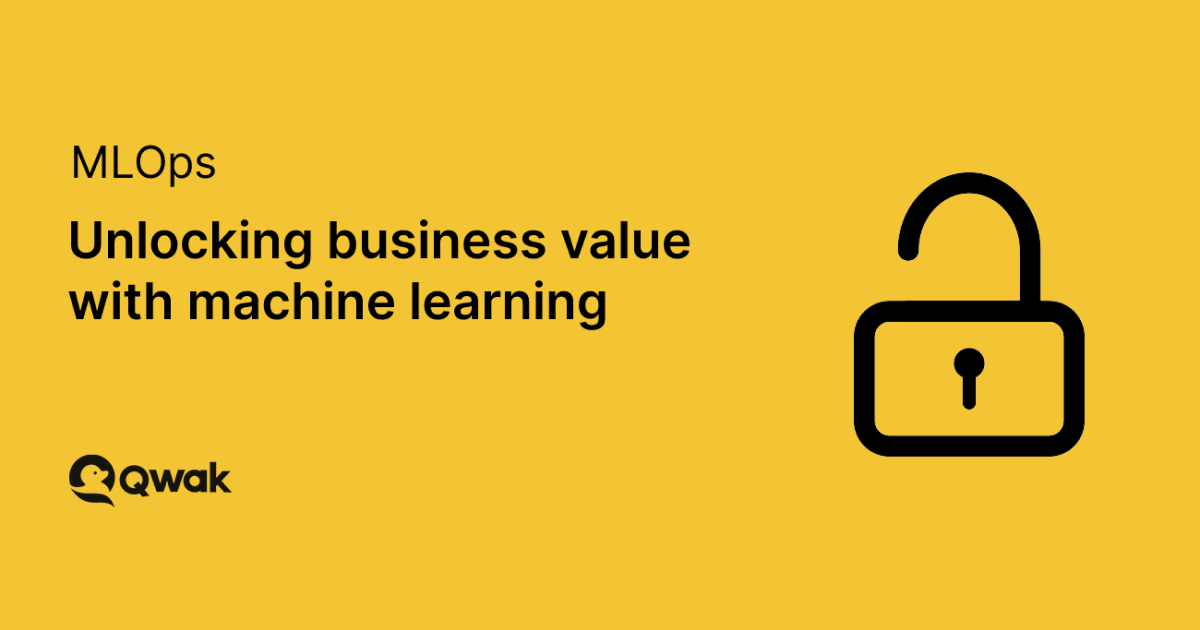
In a few hundred years, when people look back on this point in history, the emergence of AI and machine learning (ML) will be viewed as a significant milestone that changed the world.Â
Thatâs because we are living in an AI revolution, and if you are a business owner whose company isnât yet mining data and leveraging its advanced capabilities, you should seriously think about catching up before you get left behind.Â
Although ML has not yet completely transformed our lives to the extent that researchers believe it can â in fact, we are not even close â it has established itself as the best way for businesses to unlock more value from their customer data. Things like Facebookâs ability to recognize our faces and Netflixâs ability to accurately recommend new shows that interest us are both examples of its raw power.Â
And for businesses that choose to take advantage of this power, unlimited opportunities await. You only need to look at the numbers to realize that this is the case; the value of the global machine learning market was US$8 billion in 2019, and this is estimated to reach US$117 billion by the end of 2027. This represents an astonishing compound annual growth rate (CAGR) of 39 percent.Â
It is clear that organizations are beginning to pay attention, too. According to a recent report by Refinitiv, 46 percent of organizations have already deployed ML at the core of their organizations and are extracting real business value from it.
What is meant by âbusiness valueâ in machine learning?
When we talk about business value in the context of machine learning, however, we are not necessarily talking in monetary terms or about model sophistication.
Rather, business value comes down to a modelâs performance metrics and how well it solves your customersâ problems. After all, if your model performs badly and is unable to help your customers then it is going to be worth very little to you.Â
Letâs say that you are the CEO of a start-up bank that has deployed a machine learning model to assess new customers' applications according to Know Your Customer and anti-money laundering best practices. It is not enough for this model to simply say âYESâ or âNOâ and for you to base your decision-making on that; the model needs to perform well enough to give you context.
This is the basis for unlocking business value from machine learning â when you and your customers are informed, you build trust and establish a strong reputation.Â
5 tips for creating business value from machine learning
We know that itâs easy to say, âdeploy models that perform wellâ and âkeep your customers informedâ, but how can organizations go about doing this in practice?Â
Every organization is different, but there are a few things that are relevant across all industries. While this is not an exhaustive list nor is it intended to act as a guide, these tips should help you establish a strong foundation upon which you can do more to unlock business value from your ML models.Â
1. Begin with a clear business challengeÂ
One of the most common mistakes that organizations make when they start new ML projects is using their data sources and available capabilities as the starting point, rather than beginning by defining a clear problem that they want to solve.Â
While this appears to make sense on the face of it, not least because the most sophisticated ML models are powered by huge, high-quality training data, the approach of applying ML where you already have the most data doesnât tend to work on a smaller scale.Â
In our experience, it doesnât take a huge amount of next-level data to solve the challenges that most businesses want to tackle with ML models. It is more often the case that successful projects are designed and built around a specific, clearly-defined organizational challenge rather than the other way around.Â
Organizations that use their existing data as the starting point are often left with ML models that, while sophisticated, do not solve any immediate problems⊠and when you are not solving problems and overcoming challenges, you arenât getting any business value from your ML models.Â
2. Build your organizationâs data ecosystem
Although we live in a world that is driven by data, those that donât understand it will rarely give it the full respect that it deserves.Â
There are many instances of organizations not treating data as the valuable asset that it is and instead opt to regard it as a burden. That is, until the point when the inevitable data breach takes place and untold damage is done to the organization. Only then will they suddenly realize just how important and valuable their data truly is. Â
That is why one of the first and arguably most critical steps (after defining a clear business challenge) for any organization that wishes to unlock value from data and ML models is to build out a robust data ecosystem. Doing so enables seamless data management and integration across the entire organization.Â
Without an efficient and robust data ecosystem in place, valuable organizational data will simply end up being stuck in the void where it cannot be properly managed, democratized, and used to its full extent.Â
Simply put, without a solid data ecosystem, you will lack the foundation that is needed for you to make the right decisions and unlock the full value from your data.Â
3. Take the right approach to talent acquisition
One mistake we see organizations making time and time again when it comes to hiring ML talent is that they are simply looking for too much.Â
It often seems to be the case that business leaders have this idea in their head that data scientists are only worth hiring if they have PhDs, world-leading programming abilities, mind-blowing analytical skills, and are well-versed in the ins and outs of business management.
It is highly unlikely that firms will come across such people, however. While candidates with such extraordinary skillsets do exist, they are the exception to the norm, and it is unrealistic for business leaders to expect to hire a full team of these people.Â
Instead, business leaders should take an approach to talent acquisition that views ML as a team effort, thereby seeking to build a cross-functional team where every individual has one thing that they excel at. An individual data engineer, an individual statistician, an individual programmer, and an individual domain expert will be able to achieve more as a team than a single person that professes to be all of that and more (or worse, four people who do!)
4. Run test pilots of your ML model
It is important to run test pilots of your model early on to avoid so-called âpilot purgatoryâ â a term that describes the situation where a functioning ML model exists but is not being used within the organization or in the role for which it was designed. Essentially, a model sits in purgatory if it works properly â at least to an extent â yet it is not being used by anybody.Â
Pilot purgatory tends to happen because ML teams donât feel ready to deploy their model via a test pilot, usually because they feel that more needs to be added or perfected despite the fact that the model works, even if only at a basic level. However, avoiding test pilots is a big no-no because they provide ML teams with a valuable opportunity to experiment with their model prior to any large-scale quantitative research, thus reducing the potential for time and money to be wasted on an inadequate model.Â
In essence, live pilot phases provide a âtry-before-you-buyâ that helps organizational ML teams to understand the potential challenges that lay ahead with their ML model, thus enabling further design, tweaking, and optimization.Â
When designing a test pilot for an ML model, ML teams should consider the following:
- Clearly define the business outcome the ML model should deliver
- Choose the right testing approach
- Anticipate potential challenges and roadblocks and be ready to pivot
- Understand the difference between testing vs production-ready
5. Know when to change course
Organizations often calculate a detailed business case for the creation and adoption of ML models. While these are made with the best interests of the business at heart, they tend to be based on unrealistic assumptions. When decision-makers realize that these assumptions canât be met by the ML model they have designed and adopted, they often try to twist the situation to fit the original business case.
This is the wrong approach to take and only serves to put the business value of the ML model at risk. Instead, decision-makers and ML teams should be prepared to change course, whether that means acquiring new data sources, adapting business processes, or adjusting their ML model.Â
Although nobody can predict what changing course might look like or mean for your ML model and your organization, thatâs okay; ML models are not cut-and-paste exercises. They are tools that you build, optimize, and adapt to overcome challenges and maximize the value you extract from your business processes and assets.Â
Unlocking value requires real-world deployment
As we have already explored, the path to unlocking business value from ML models is far from straightforward. You will therefore want to implement a process that not only measures and optimizes models during the pre-production process but also ensures continuous improvement in production.
A continuous improvement process works by gathering additional information from your working model as it interacts with your users and your wider IT ecosystem, which improves the likelihood of good decisions over time through ongoing measuring, learning, and re-optimization. Â
âDeveloping machine learning applications is an iterative process, requiring experimentation. If your organizational culture doesnât encourage experimentation or if it treats failure (AKA learning) as something to be avoided at all costs, then this will be a significant barrier to applying ML effectively.â â Michelle Lee, former VP of Amazon Machine Learning Solutions Lab and Computer Vision.Â
Conclusion
We are living amid an AI revolution. In recent years, there has been a massive tectonic shift in the business landscape with the emergence of machine learning for creating business value through problem-solving, predictive models, deeper insights, and better customer experience.Â
It is hardly surprising then that pretty much every organization that wants to survive through to tomorrow is putting huge effort into building its own ML solutions.Â
All too often, however, organizations kick off their ML projects with the misconception that they need to start with their data and build an innovative piece of technology around it. This is the wrong approach.Â
What is instead required is a full and solid understanding of the problem that the organization is trying to solve. From here, organizations can begin to build out their data ecosystems, incorporate human factors, and put together a model that can solve this problem so that real, tangible business value can be derived from it.Â
While some organizations approach the ML challenge alone, the majority turn to full-service data and ML engineering platforms like Qwak that have the expert knowledge, infrastructure, and capacity in place to help organizations unlock business value from their ML models and data without having to invest huge amounts of time or money.Â
Want to find out more about how Qwak could help you deploy your ML models effectively and efficiently? Get in touch for your free demo!